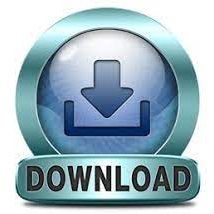
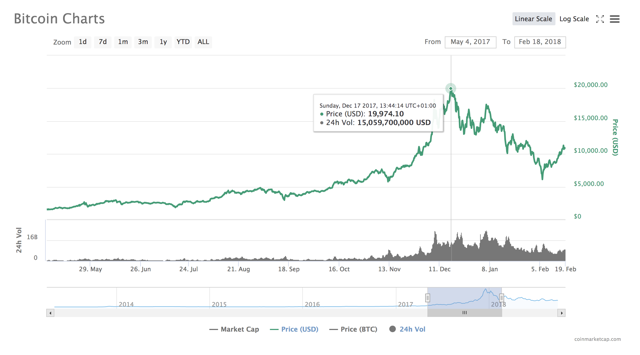
Econ Lett 130: 32–36.ĭwyer GP (2015) The economics of Bitcoin and similar private digital currencies. doi: 10.1016/j.intfin.2015.02.010Ĭheah ET, Fry J (2015) Speculative bubbles in Bitcoin markets? An empirical investigation into the fundamental value of Bitcoin.
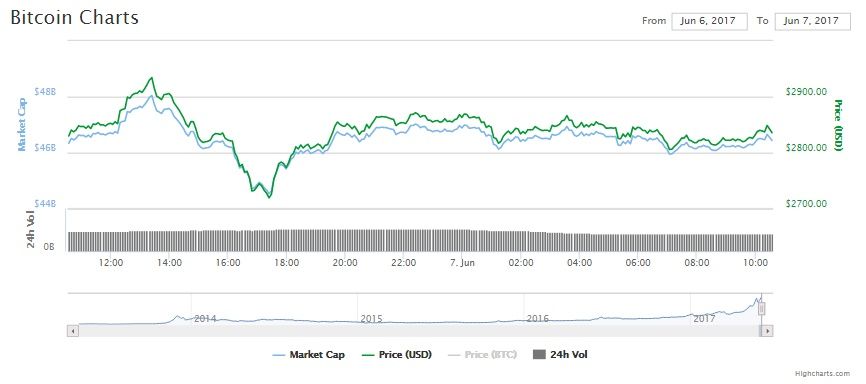
(2015) Price discovery on Bitcoin exchanges. Comput Fraud Security 2013: 5–8.īrandvold M, Molnár P, Vagstad K, et al. (2017b) On the hedge and safe haven properties of Bitcoin: Is it really more than a diversifier? Financ Res Letters 20: 192–198.īradbury D (2013) The problem with Bitcoin.

Econ 11: 1–16.īouri E, Molnár P, Azzi G, et al. īouri E, Azzi G, Dyhrberg AH (2017a) On the return-volatility relationship in the Bitcoin market around the price crash of 2013. (2017) high frequency: Tools for High frequency Data Analysis. doi: 10.1093/jjfinec/nbh001īlau BM (2017) Price dynamics and speculative trading in bitcoin. (2017) Some stylized facts of the Bitcoin market, Physica A: Stat Mechanics Appl 484: 82–90.īarndorff-Nielsen OE, Shephard N (2004) Power and Bipower Variation with Stochastic Volatility and Jumps.
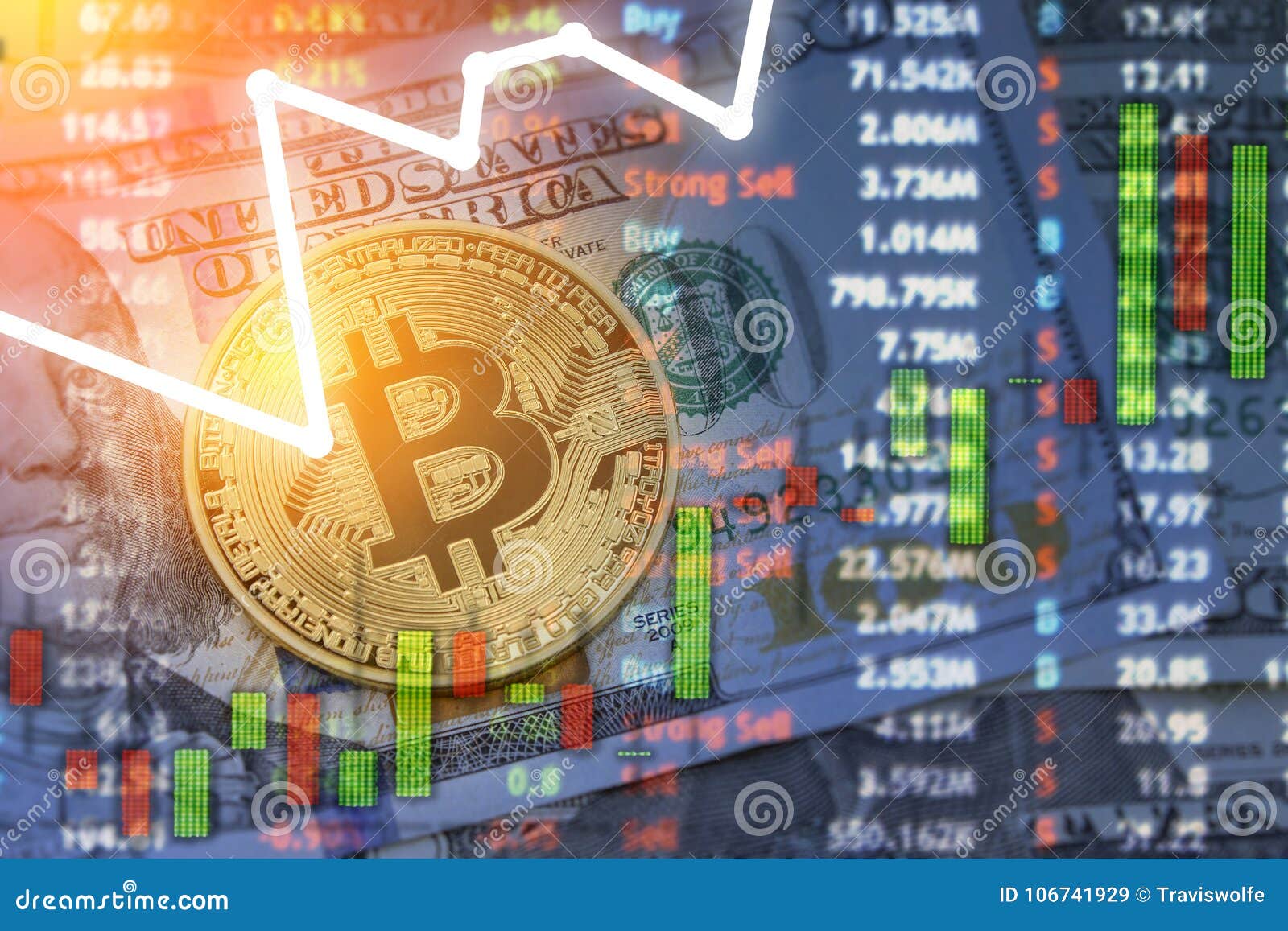
Econ Model 64: 74–81.īariviera AF, Basgall MJ, Hasperué W, et al. (2017) Can volume predict Bitcoin returns and volatility? A quantiles-based approach. doi: 10.1016/S0304-405X(01)00055-1Īndersen TG, Bollerslev T, Diebold FX (2007) Roughing it up: including jump components in measuring, modeling and forecasting asset return volatility. (2001) The distribution of realized stock return volatility. (2000) Exchange Rate Returns Standardized by Realized Volatility are (Nearly) Gaussian. Volatility Analysis of Bitcoin Price Time Series. The results show that such an artificial neural network prediction is capable of approximate capture of the actual log return distribution more sophisticated methods, such as recurrent neural networks and LSTM (Long Short Term Memory) techniques from deep learning may be necessary for higher prediction accuracy.Ĭitation: Lukáš Pichl, Taisei Kaizoji. Finally, a feed-forward neural network with 2 hidden layers using 10-day moving window sampling daily return predictors is applied to estimate the next-day logarithmic return. (2007) applies reasonably well to the BTCUSD dataset. In this work we demonstrate that the Heterogeneous Autoregressive model for Realized Volatility Andersen et al. The volatility of BTC exchange rates is modeled using the day-to-day distribution of logarithmic return, and the Realized Volatility, sum of the squared logarithmic returns on 5-minute basis. Whereas the arbitrage spread for EUR-USD currency pair is found narrow at the order of a percent, at the 1 hour sampling period the arbitrage spread for USD-CNY (and similarly EUR-CNY) is found to be more substantial, reaching as high as above 5 percent on rare occasions. Arbitrage opportunities are studied using the EUR, USD and CNY currencies. Distribution of trading volumes (1 sec, 1 min, 1 hour and 1 day) aggregated from the Kraken BTCEUR tick data is provided that shows the artifacts of algorithmic trading (selling transactions with volume peaks distributed at integer multiples of BTC unit). Multi-scale analysis is performed from the level of the tick data, through the 5 min, 1 hour and 1 day scales. The average day-to-day return throughout this period is 0.328%, amounting in exponential growth from 6 USD to over 4,000 USD per 1 BTC at present. In this work we focus on the price of Bitcoin in terms of standard currencies and their volatility over the last five years. Bitcoin has the largest share in the total capitalization of cryptocurrency markets currently reaching above 70 billion USD.
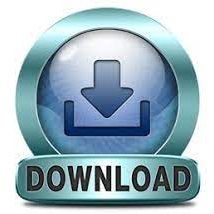